Ideal Tips About How To Fix Multicollinearity
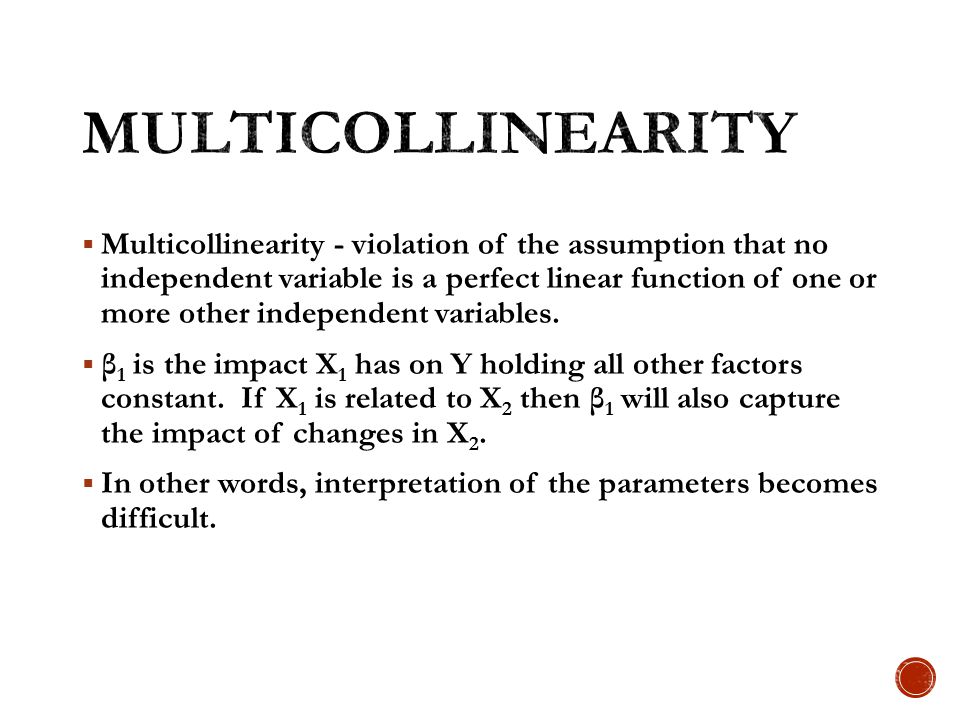
Now we run a multiple regression analysis using spss.
How to fix multicollinearity. You may use ridge regression or principal component regression or partial least squares regression. If you determine that you do need to fix multicollinearity, then some common solutions include: If you have two or more factors with a high vif,.
Remove one or more of the highly correlated variables. The best solution for dealing with multicollinearity is to understand the cause of multicollinearity and remove it. Using example data, we calculate and interpret correlation coefficients and varianc.
In this article, we will see how to find multicollinearity in data using correlation matrix and pca, and. This video explains the concept of multicollinearity in a multiple regression model.the video explains how to detect multicollinearity in e views and how to. Correcting multicollinearity remove one of highly correlated independent variable from the model.
In the r custom function below, we are removing the variables with the largest vif until all variables. Are some of the techniques or hacks to find multicollinearity in the data. Variable selection the most straightforward method is to remove some variables that are highly correlated to others and.
You can use the python library modelauto to solve muticoliniarity easily. Multicollinearity occurs because two (or more) variables are related or they. Here is the code and its result for doing.
The condition number assesses the multicollinearity for an entire model rather than individual terms. All the variables having vif higher than 2.5 are faced with a problem of multicollinearity. There are multiple ways to overcome the problem of multicollinearity.